Author: Chris Davison, University of Strathclyde
Wheat is a worldwide staple crop, estimated to be 3rd in yield behind corn and rice (link). Because of its importance to the nutrition of billions of people around the globe, it’s widely studied. Significant progress has been made in farming operations, utilising machinery to reduce the labour required to plant and harvest, while different varieties of wheat have been bred to adapt to different environmental situations--some ‘rugged’ varieties are more suited to lower quality soil and produce a more consistent yield, while other varieties are more sensitive yet can yield much more in optimum conditions.
In order to meet the demands of the food supply, farmers must monitor the growth of their crops. Traditionally, this has been accomplished through manually doing counts of wheat ears on small patches of a crop--a post is jammed into the ground with a square box, and any ears that come up through the box are counted (Figure 1).
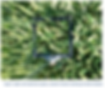
From these counts, an estimate of the yield of the entire field can be calculated. With differences in soil quality, nutrient uptake, rainfall, and sunlight, there are many opportunities for error when attempting to extrapolate from these small samples.
We at the University of Strathclyde, as part of the Horizon2020 CYBELE project (link), are utilising Deep Learning to automatically detect wheat ears in photos from consumer-level devices (other approaches have utilised thermal imagery, where you can more readily detect heat stored in the wheat ear, however these devices are much more expensive and thus less appealing to farmers). Mikołaj Czerkawski has led development of a model using publicly available data (link). This dataset provided images of wheat crops from around the world, along with the precise location of the ears within each image. This lets us train an algorithm to detect the wheat ears in new images. We further tested this model by gathering our own samples--capturing images using consumer-level GoPro cameras, and counting manually--which the model has no prior information of the location of the ears within the image. Figure 2 shows an example of these detections, with each wheat ear surrounded by a red box.

This detection of wheat ears can be applied to any new photo captured, and by removing the labour required to manually count the ears, time can instead be used to capture more samples, and thus get a much more representative measure of the growth of the field and the expected yield.
In the future, it will be possible to utilise drones to automate this entirely, allowing farmers to count the yield across the entirety of their farm. With knowledge of the height of the drone during flight, and the characteristics of the onboard camera, the field of view can be calculated, which then allows a crop density and yield calculation. Entirely automating the process would make it much easier for farmers to count the yield of their crop multiple times throughout the growth cycle, including variations within the same field. With this insight, farmers can better target the application of fertilisers (which reduces customer environmental concerns), as well as more accurately predict when a crop may be ready for harvest.
Author:
Chris Davison, Research Assistant, University of Strathclyde